- Prompt Hunt Newsletter
- Posts
- AI, Twitter, and Misinformation
AI, Twitter, and Misinformation
Moderating the town square
Here's your daily briefing:
Here's an interesting coupla tweets (and blog post) about Elon Musk's decision to fire the META team (ML, Ethics, Transparency, Accountability) at Twitter and how this might affect the bird app's ability to fight AI-generated misinformation:
Nov 7: Musk says his mission is to make Twitter the "most accurate source of information." Three days earlier, Nov 4: Musk fires the META team at Twitter, in charge of responsible AI.
He shouldn't be the face of our town square of reliable information:
— Alberto Romero (@Alber_RomGar)
8:10 PM • Nov 8, 2022
Generating misinformation is easier, cheaper, and faster than ever. Anyone can exploit AI systems: from conventional deepfakes to language models like GPT-3 and text-to-image diffusion models like Stable Diffusion. These AIs can’t tell right from wrong and often make up realities that have no grounding in the real world. At the same time, because these systems evolve so fast, we’re lagging behind in detection and recognition countermeasures.
And I’d add here another problem on top of this. Recommender systems, which literally decide what we see on Twitter (or any other social media app), are also driven by opaque AI algorithms. This wouldn’t be much of a problem if it wasn’t for Musk’s recent decisions: first, he decided to remove identity verification, and then he fired the team in charge of algorithmic responsibility.
if you are serious @elonmusk about trying to make Twitter the most accurate source of information, we should talk. And you need to start by understanding the core technical issue:
— Gary Marcus (@GaryMarcus)
1:34 AM • Nov 7, 2022
This "PaperCut" model for Stable Diffusion is very cool:
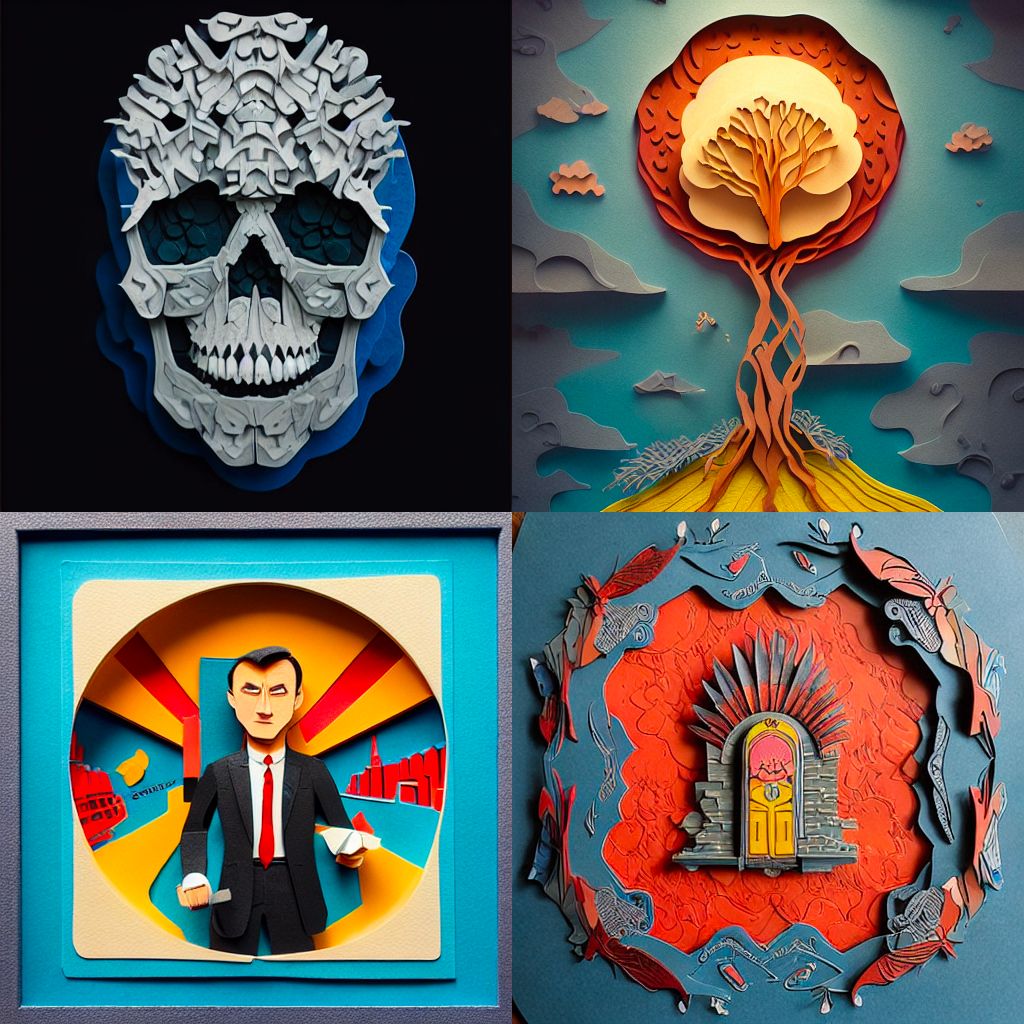

Researchers at MIT created an algorithm that tackles the issue of AI being too “curious” and getting preoccupied by the task at hand. Their algorithm automatically dials up the curiosity as needed, and dampens it if the agent has got enough supervision from the environment to get the "job" done.
New algorithm overcomes the problem of AI being too “curious,” utilizing intrinsic rewards when they are helpful to save practitioners' time on deciding which algorithm to use on any new task: bit.ly/3fStdhe
— MIT CSAIL (@MIT_CSAIL)
6:26 PM • Nov 9, 2022
Just like the dilemma faced by humans in selecting a restaurant, these [AI] agents also struggle with balancing the time spent discovering better actions (exploration) and the time spent taking actions that led to high rewards in the past (exploitation). Too much curiosity can distract the agent from making good decisions and too little means the agent will never discover good decisions.
In this Stanford HAI (Human-Centered Artificial Intelligence) article, Nikki Goth Itoi discusses the question of data-privacy in relation to ever-increasingly intelligent (and nosy) smart assistants:
Many of us use smart assistants on our phones and in our homes. How can we be assured that our personal information is kept private when these machine learning models answer our questions? stanford.io/3hsumN7
— Stanford HAI (@StanfordHAI)
5:11 PM • Nov 9, 2022
If we expect digital assistants to facilitate personal tasks that involve a mix of public and private data, we’ll need the technology to provide “perfect secrecy,” or the highest possible level of privacy, in certain situations. Until now, prior methods either have ignored the privacy question or provided weaker privacy guarantees.
Check out this blog post and Youtube video by Gateway about how he used Stable Diffusion Infinity outpainting to create a very large mural:
Gotta catch em' all:
AI-Generated Pokémon Evolution
— Max Woolf (@minimaxir)
11:09 PM • Nov 8, 2022
Especially this one 😝 🤣:
I like the one where he's briefly a ballchinian version of Purugly and Pikachu
— Ryan Mather 🌿✨ (@Flomerboy)
11:19 PM • Nov 8, 2022
Large language models are magical. But using them in production has been tricky, until now.
I’m excited to share ✨Spellbook🪄✨— the platform for LLM apps from @scale_AI. 🧵
— Alexandr Wang (@alexandr_wang)
8:40 PM • Nov 8, 2022
Here's another piece along the same lines as the one we shared yesterday about AI bias, this one from Stanford HAI/Mutale Nkonde:
Sociologist @mutalenkonde warns that the AI behind much of today’s social media is inherently biased—but it’s not too late to do something about it.
— Stanford HAI (@StanfordHAI)
4:01 PM • Nov 9, 2022
Nice informative thread from Assembly AI on 10 AI terms you should know:
Here are 10 common AI terms explained in an easily understandable way.
1. Classification
2. Regression
3. Underfitting
4. Overfitting
5. Cost function
6. Loss function
7. Validation data
8. Neural Network
9. Parameter
10. HyperparameterAI Thread🧵👇
— AssemblyAI (@AssemblyAI)
3:52 PM • Nov 8, 2022

Take us away, GPT-3:
